The hype around DeepMind’s new AI model misses what’s actually cool about it
Some worry that the chatter about these tools is doing the whole field a disservice.
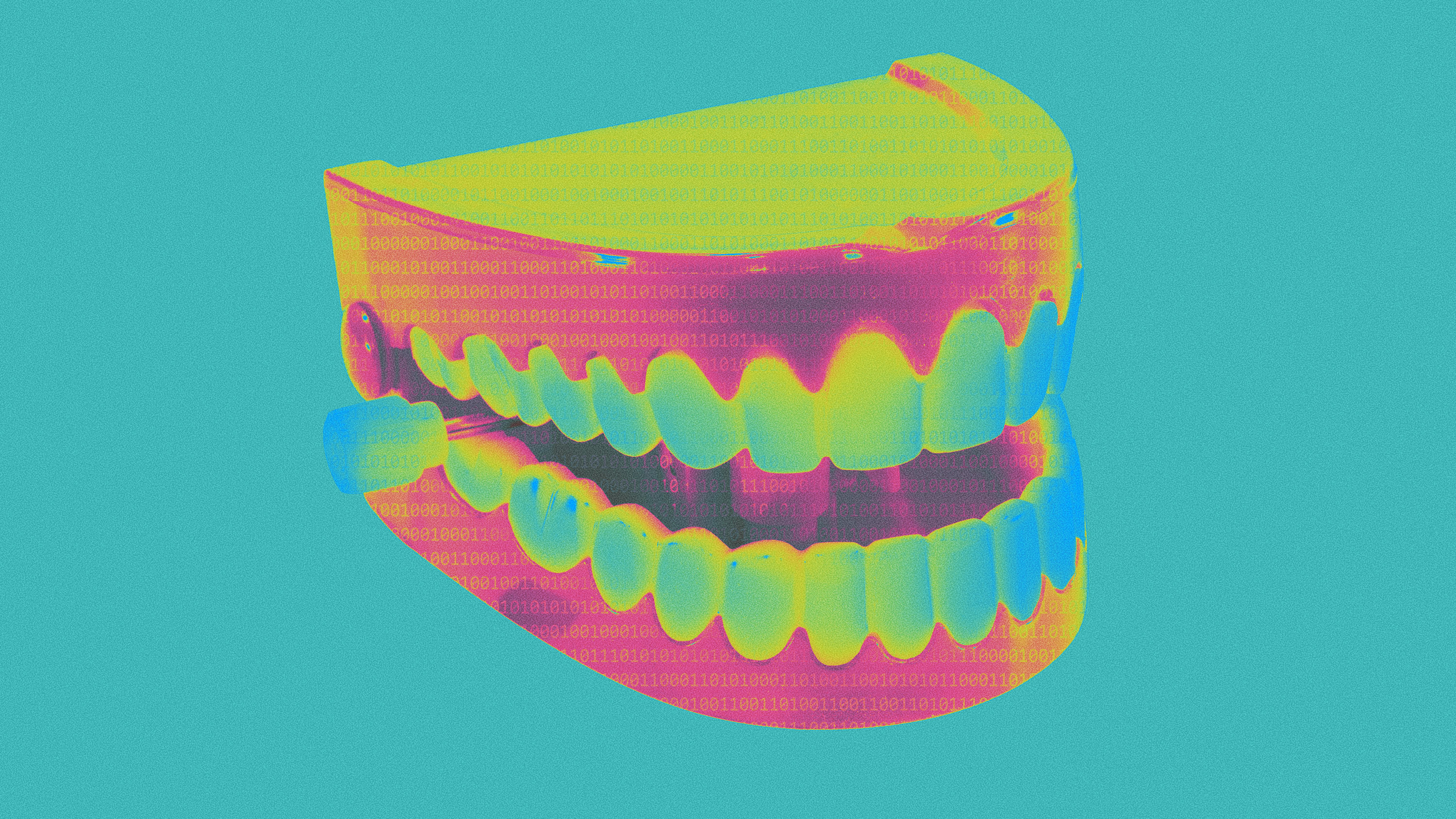
Earlier this month, DeepMind presented a new “generalist” AI model called Gato. The model can play Atari video games, caption images, chat, and stack blocks with a real robot arm, the Alphabet-owned AI lab announced. All in all, Gato can do 604 different tasks.
But while Gato is undeniably fascinating, in the week since its release some researchers have gotten a bit carried away.
One of DeepMind’s top researchers and a coauthor of the Gato paper, Nando de Freitas, couldn’t contain his excitement. “The game is over!” he tweeted, suggesting that there is now a clear path from Gato to artificial general intelligence, or AGI, a vague concept of human- or superhuman-level AI. The way to build AGI, he claimed, is mostly a question of scale: making models such as Gato bigger and better.
Unsurprisingly, de Freitas’s announcement triggered breathless press coverage that DeepMind is “on the verge” of human-level artificial intelligence. This is not the first time hype has outstripped reality. Other exciting new AI models, such as OpenAI’s text generator GPT-3 and image generator DALL-E, have generated similarly grand claims. For many in the field, this kind of feverish discourse overshadows other important research areas in AI.
That’s a shame, because Gato is an interesting step. Some models have started to mix different skills: DALL-E, for example, generates images from text descriptions. Others use a single training technique to learn to recognize pictures and sentences. And DeepMind's AlphaZero learned to play Go, chess, and shogi.
But here’s the crucial difference: AlphaZero could only learn one task at a time. After learning to play Go, it had to forget everything before learning to play chess, and so on. It could not learn to play both games at once. This is what Gato does: it learns multiple different tasks at the same time, which means it can switch between them without having to forget one skill before learning another. It’s a small advance but a significant one.
The downside is that Gato doesn’t perform the tasks as well as models that can only do one thing. Robots still need to learn “common-sense knowledge” about how the world works from text, says Jacob Andreas, an assistant professor at MIT who specializes in artificial intelligence and natural-language and speech processing.
This could come in handy in robots that could help people around the house, for example. “When you drop [a robot] into a kitchen and ask them to make a cup of tea for the first time, they know what steps are involved in making a cup of tea and in which cabinet tea bags are likely to be located,” says Andreas.
Some external researchers were explicitly dismissive of de Freitas’s claim. “This is far from being ‘intelligent,’” says Gary Marcus, an AI researcher who has been critical of deep learning. The hype around Gato demonstrates that the field of AI is blighted by an unhelpful “triumphalist culture,” he says.
The deep-learning models that often generate the most excitement about the potential to reach human-level intelligence make mistakes that “if a human made these errors, you’d be like, something’s wrong with this person,” Marcus says.
“Nature is trying to tell us something here, which is this doesn’t really work, but the field is so believing its own press clippings that it just can’t see that,” he adds.
Even de Freitas’s DeepMind colleagues Jackie Kay and Scott Reed, who worked with him on Gato, were more circumspect when I asked them directly about his claims. When asked whether Gato was heading toward AGI, they wouldn’t be drawn. “I don’t actually think it’s really feasible to make predictions with these kinds of things. I try to avoid that. It’s like predicting the stock market,” said Kay.
Reed said the question was a difficult one: “I think most machine-learning people will studiously avoid answering. Very hard to predict, but, you know, hopefully we get there someday.”
In a way, the fact that DeepMind called Gato a “generalist” might have made it a victim of the AI sector’s excessive hype around AGI. The AI systems of today are called “narrow,” meaning they can only do a specific, restricted set of tasks such as generate text.
Some technologists, including some at DeepMind, think that one day humans will develop “broader” AI systems that will be able to function as well as or even better than humans. Though some call this artificial general intelligence, others say it is like "belief in magic.“ Many top researchers, such as Meta’s chief AI scientist Yann LeCun, question whether it is even possible at all.
Gato is a “generalist” in the sense that it can do many different things at the same time. But that is a world apart from a “general” AI that can meaningfully adapt to new tasks that are different from what the model was trained on, says MIT’s Andreas: “We’re still quite far from being able to do that.”
Making models bigger will also not address the issue that models don’t have “lifelong learning,” which would mean that if taught something once, they would understand all the implications and use it to inform all the other decisions they make, he says.
The hype around tools like Gato is harmful for the general development of AI, argues Emmanuel Kahembwe, an AI and robotics researcher and part of the Black in AI organization cofounded by Timnit Gebru. “There are many interesting topics that are left to the side, that are underfunded, that deserve more attention, but that’s not what the big tech companies and the bulk of researchers in such tech companies are interested in,” he says.
Tech companies ought to take a step back and take stock of why they are building what they are building, says Vilas Dhar, president of the Patrick J. McGovern Foundation, a charity that funds AI projects “for good.”
“AGI speaks to something deeply human—the idea that we can become more than we are, by building tools that propel us to greatness,” he says. “And that’s really nice, except it also is a way to distract us from the fact that we have real problems that face us today that we should be trying to address using AI.”
Deep Dive
Artificial intelligence
Google DeepMind used a large language model to solve an unsolved math problem
They had to throw away most of what it produced but there was gold among the garbage.
Unpacking the hype around OpenAI’s rumored new Q* model
If OpenAI's new model can solve grade-school math, it could pave the way for more powerful systems.
Finding value in generative AI for financial services
Financial services firms have started to adopt generative AI, but hurdles lie in their path toward generating income from the new technology.
Google DeepMind’s new Gemini model looks amazing—but could signal peak AI hype
It outmatches GPT-4 in almost all ways—but only by a little. Was the buzz worth it?
Stay connected
Get the latest updates from
MIT Technology Review
Discover special offers, top stories, upcoming events, and more.