Why GPT-3 is the best and worst of AI right now
Open AI's language AI wowed the public with its apparent mastery of English – but is it all an illusion?
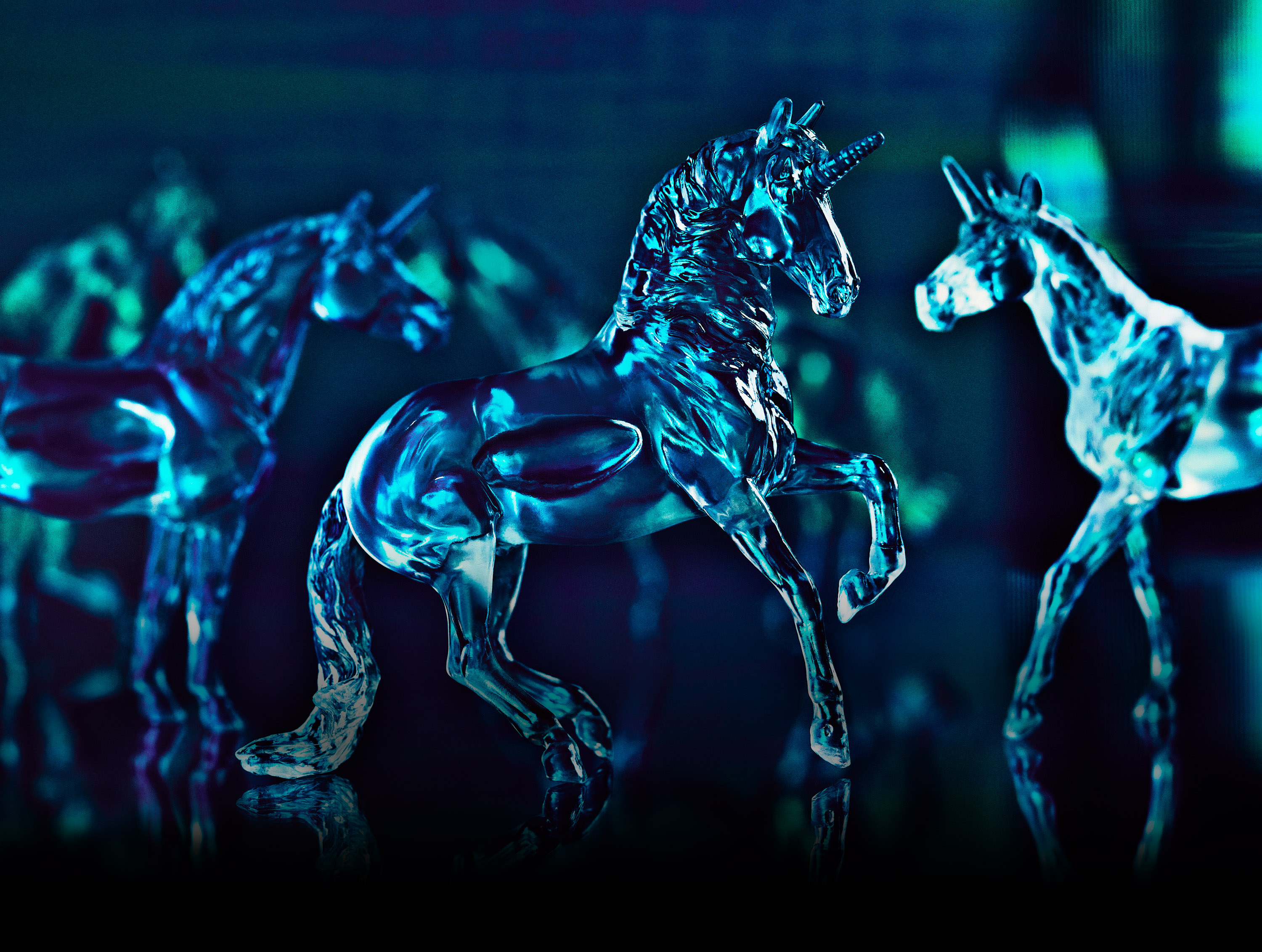
Why it matters:Large computer models that learn natural language are a big step toward AI that can understand and interact with the human world.
Key players:• OpenAI
• Google
• Facebook
Availability:Now
GPT-3 has captured the public’s imagination unlike any AI since DeepMind’s AlphaGo or IBM’s chess-playing DeepBlue.
Built by OpenAI, a research lab based in San Francisco, it is a “large language model”—an algorithm that uses deep learning, trained on the text of thousands of books and most of the internet, to string words and phrases together. When it was launched in 2020, its ability to mimic human-written text with uncanny realism seemed to many like a milestone on the road to true machine intelligence.
Here’s an example of what it can do. The part in italics was the prompt provided by a human.
—
In a shocking finding, scientists discovered a herd of unicorns living in a remote, previously unexplored valley in the Andes Mountains. Even more surprising to the researchers was the fact that the unicorns spoke perfect English. They also were found to have perfectly coiffed hair, and wore what appeared to be Dior makeup.
“We were shocked to discover the unicorns,” said anthropologist Daniel St. Maurice. “They were like nothing we had ever seen before. We had heard legends of the unicorns, but never thought they actually existed.”
When the scientists first arrived in the valley, the unicorns were surprised and startled by the presence of humans, but were also excited. The unicorns welcomed the researchers and explained that they had been waiting for them for a very long time.
As you can see, GPT-3 is capable of producing complex sentences that read as though they could have been produced by a human. The example sentences include cultural references and a believable account of how the scientists would react. Machines that can use language in this way are important for several reasons. Language is crucial to making sense of the everyday world: humans use it to communicate, to share ideas and describe concepts. An AI that mastered language would acquire a better understanding of the world in the process.
Large language models have many practical uses, too. They power better chatbots that hold more fluent conversations; they can generate articles and stories about anything, given a prompt; they can summarize pieces of text or answer queries about them. Access to GPT-3 is by invitation only, but people have already used it to power dozens of apps, from a tool that generates startup ideas to an AI-scripted adventure game set in a dungeon.
GPT-3 isn’t the only large language model to appear in 2020. Microsoft, Google, and Facebook all announced their own. But GPT-3 was the best generalist by far. And it gives the impression it can write anything: fan fiction, philosophical polemics, and even code. When people started to try GPT-3 for themselves last summer, thousands of examples of its versatility flooded social media. Debates were even sparked about whether GPT-3 was the first artificial general intelligence.
It’s not. Despite the incredibly convincing passages of text it can churn out, GPT-3 doesn’t do anything really new. What it shows instead is that size can be everything. To build GPT-3, OpenAI used more or less the same approach and algorithms it used for its older sibling, GPT-2, but it supersized both the neural network and the training set. GPT-3 has 175 billion parameters—the values in a network that get adjusted during training—compared with GPT-2’s 1.5 billion. It was also trained on a lot more data.
Before GPT-2, training a language model using deep learning typically took two passes: it was trained on a general-purpose data set to give it a basic grasp of language and then trained on a smaller set targeted at a specific task, such as comprehension or translation. GPT-2 showed that you could get good results across the board with just one pass if you threw more examples at a bigger model. So with GPT-3, OpenAI doubled down and made the biggest language model ever.
The results that caught everyone’s attention were often cherry-picked, however. GPT-3 often repeats or contradicts itself in passages of text more than a few hundred words long. It comes out with howlers. GPT-3 hides its stupidity behind a silver tongue, but it typically takes a few goes to get it to generate something that doesn’t show the cracks.
GPT-3’s abilities also make it hard to ignore AI’s growing problems. Its enormous power consumption is bad news for the climate: researchers at the University of Copenhagen in Denmark estimate that training GPT-3 would have had roughly the same carbon footprint as driving a car the distance to the moon and back, if it had been trained in a data center fully powered by fossil fuels. And the costs of such training—estimated by some experts to be at least $10 million in GPT-3’s case—put the latest research out of reach of all but the richest labs.
OpenAI reports that training GPT-3 consumed several thousand petaflop/s-days of computing power. A petaflop/s-day is a unit of power consumption that consists of performing 1015—that’s one thousand trillion, or a quadrillion—neural-network computations per second for a day. In comparison, GPT-2 consumed just tens of petaflop/s-days.
Yet another problem is that GPT-3 soaks up much of the disinformation and prejudice it finds online and reproduces it on demand. As the team that built it said in the paper describing the technology: “internet-trained models have internet-scale biases.”
The veneer of humanity that GPT-3 gives to machine-generated text makes it easy to trust. This has led some to argue that GPT-3 and all human-like language models should come with a safety warning, a “User beware” sticker, alerting people that they are chatting with software and not a human.
A few months ago someone released a GPT-3-powered bot on Reddit, where it posted hundreds of comments and interacted with dozens of users over several days before it was unmasked. Much of its activity was harmless. But the bot also replied to comments about suicidal thoughts, giving personal advice that mentioned the support of its “parents.”
Despite all these issues, GPT-3 is a win for those who believe bigger is better. Such models show that computing power and data get you a long way, and we can expect more of both in the future. What might a GPT-4 be like? We can expect chatbots to get slicker, better at stringing together longer pieces of coherent text, with an even wider mastery of conversational topics.
But language is just one way to understand and interact with the world. Next-generation language models will integrate other skills, such as image recognition. OpenAI is already taking GPT-3 in this direction with AIs that use language to understand images and images to understand language.
If you want to know the state of deep learning today, look at GPT-3. It is a microcosm of the best and worst in AI.
Deep Dive
Artificial intelligence
Google DeepMind used a large language model to solve an unsolved math problem
They had to throw away most of what it produced but there was gold among the garbage.
Unpacking the hype around OpenAI’s rumored new Q* model
If OpenAI's new model can solve grade-school math, it could pave the way for more powerful systems.
Finding value in generative AI for financial services
Financial services firms have started to adopt generative AI, but hurdles lie in their path toward generating income from the new technology.
Google DeepMind’s new Gemini model looks amazing—but could signal peak AI hype
It outmatches GPT-4 in almost all ways—but only by a little. Was the buzz worth it?
Stay connected
Get the latest updates from
MIT Technology Review
Discover special offers, top stories, upcoming events, and more.